Sensor Fusion: Building the Bigger Picture of Risk
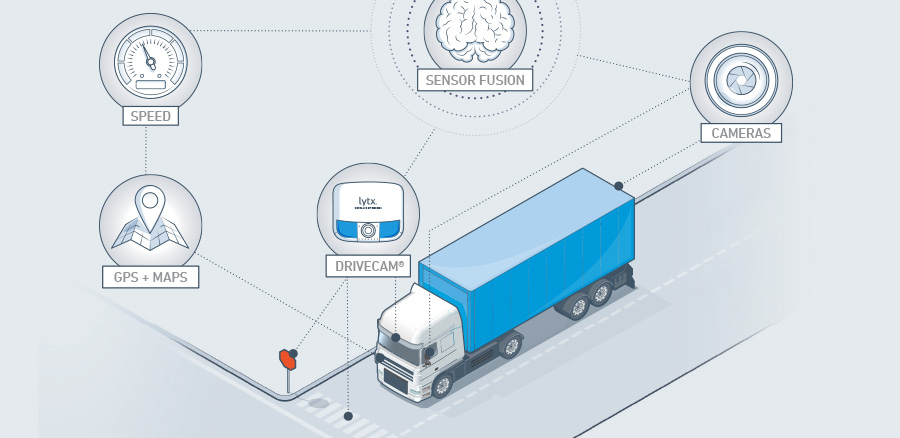
More than the sum of its parts, sensor fusion combines data from multiple sources in a way that provides better information than would be possible if these sources were used individually.
Sensor fusion is more than just the simultaneous use of multiple sensors; it combines sensor data from multiple sources in a way that provides better information than would be possible if these sources were used individually. You are already experiencing sensor fusion when you use multiple senses (sight, hearing, and touch together, for example) to perceive what is going on in the world. It is the fusing of information from these different senses that gives you a comprehensive working model of reality.
One of the most common uses for sensor fusion technology is in something you probably have with you right now: your mobile phone uses combined data from a magnetometer, accelerometer, and gyroscope together to detect motion and position.
Different sensor types have different strengths, and a single sensor can’t capture everything. By using different sensors and combining their information, we can build rich context. For example: a camera designed to record the visible spectrum can detect lane markers, stop signs, and other objects that rely on color detection, but is less effective in fog or darkness. RADAR can’t detect color, but can locate objects regardless of the weather or the presence of visible light. Combining data from both sensors provides greater accuracy and certainty of information.
The goal of sensor fusion is to reveal the bigger picture, building inferences about the real world to improve safety by reducing risk. Fused information can be fed to a neural network that learns based on analyzing combinations of sensor data including machine vision. As the neural network gains experience, it is better able to make accurate predictions about the state of the environment based on signals—sensor input combinations that indicate specific circumstances—and ignore the background noise of meaningless input combinations or inaccurate sensor readings.
In the automotive world, sensors can be used to detect lane position, steering wheel angle, acceleration, and even the driver’s head angle. Sensor data, including machine vision, is fed into a neural network that can draw conclusions about driver behavior.
Today, Lytx DriveCam® technology can detect a rolling stop at a stop sign by combining machine vision with data from the GPS, accelerometer, and the vehicle’s on-board computers. It will soon be possible to detect more subtle driver behaviors such as drowsiness by combining data from lane position and steering wheel angle sensors with machine vision that detects such cues as the driver’s head motion. Sensor fusion and artificial intelligence will continue to grow in sophistication, enabling new applications based on awareness of contexts such as terrain and weather.
To develop accurate predictive models, Lytx® combines data from multiple sensors with existing data including maps, third-party environmental information, and the results of human review of driver behavior from video. By comprehensively analyzing these multiple data sources together, Lytx improves the volume, variety, velocity, and veracity of the data set that goes into the development of the predictive model by the neural network. This creates very strong potential to detect and help prevent risky behavior, helping to change the way drivers act and interact in their work environments.