Machine Vision + Artificial Intelligence: Transportation's Fast Lane to Innovation
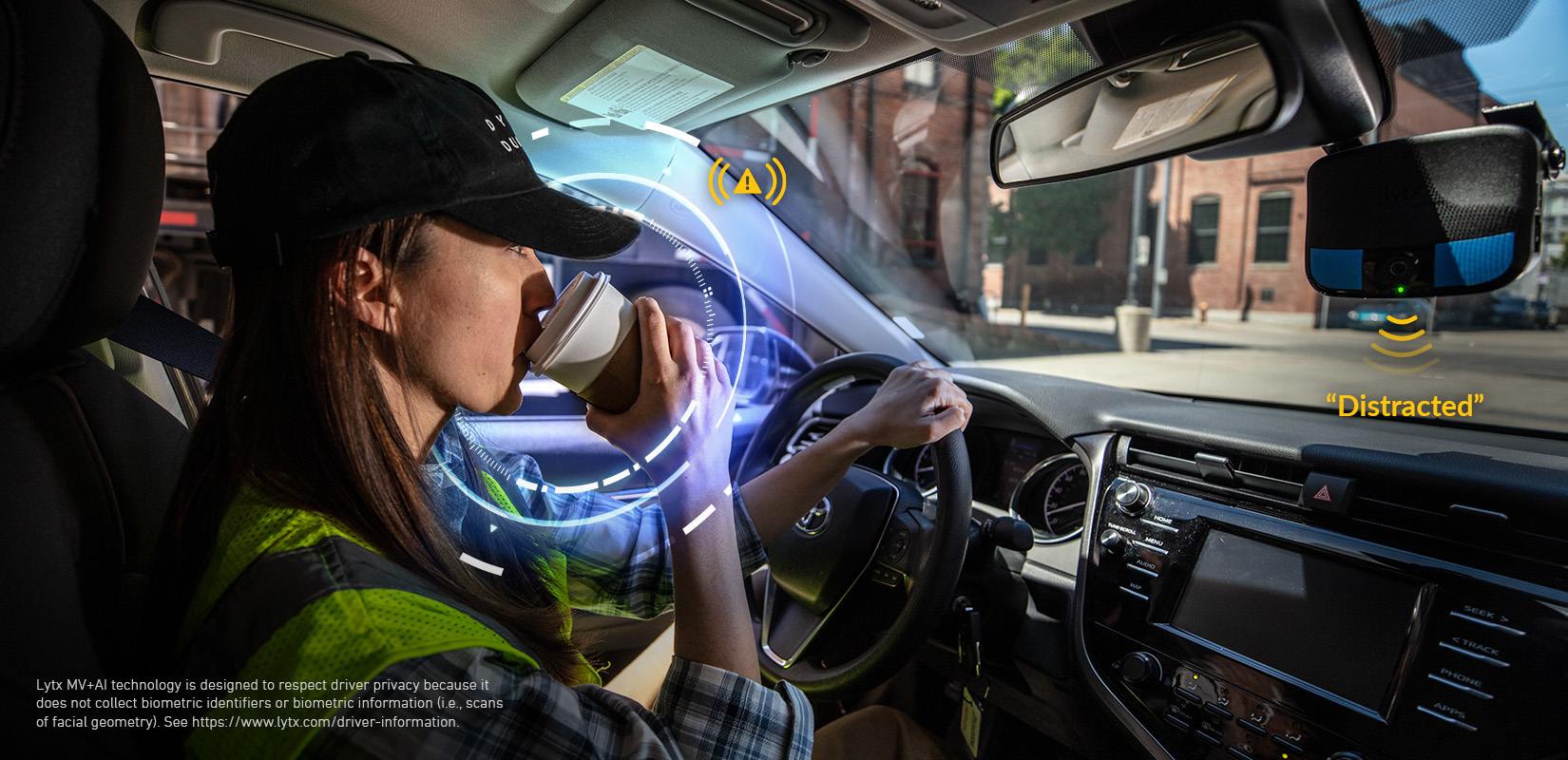
Imagine a world where all vehicles are equipped with technology that allows them to “see” the world around them. Such technology could serve as an extra pair of eyes for the driver, scanning the environment for hazards, and alerting him or her to potential collisions.
It might even proactively help the driver make evasive maneuvers, particularly in situations where slivers of seconds can mean the difference between life and death. In that world, the technology in the vehicle serves as a guardian, a sentry that’s always vigilant, always watching out for the driver and everyone around it.
The technology that promises such a future is the combination of machine vision and artificial intelligence. Machine vision (MV) confers the ability to see and recognize objects and human behavior using images, while artificial intelligence (AI) includes all types of machine intelligence such as collision detection, risky driving behavior detection, and voice recognition.
Together, MV+AI is meant to cast its gaze upon the world, understand what it sees, and anticipate what could happen next — much like how humans observe and process things in real time. And, like people, modern artificial intelligence algorithms are capable of learning and becoming more sophisticated and more accurate with its predictions as it acquires more data.
This scenario is not as far-fetched as it sounds. Already, applications of machine vision and artificial intelligence exist in manufacturing, health care, and transportation.
At Lytx, for example, researchers have developed a suite of MV+AI technologies, built specifically for commercial-driving applications. Integrated with a video telematics platform, these technologies can sense when drivers fail to stop at intersections, weave within or depart from their lanes, or follow another vehicle too closely — all behaviors that have strong correlations with collisions.
The combination of machine vision and artificial intelligence is the driving force behind these capabilities. Take for example the ability to detect whether a driver has made a complete stop at intersections with stop signs. Artificial intelligence algorithms monitor GPS location and municipal map data to detect when a vehicle is approaching an intersection. As the vehicle approaches an intersection, the AI kicks off machine vision capabilities to “look” for a stop sign. If it sees one, the algorithm then checks the engine control unit and accelerometer to determine whether the vehicle comes to a complete stop.
This is just a start. Scientists and engineers at Lytx® are rapidly developing MV+AI technologies that can detect when a driver is using a cell phone or is distracted by another handheld device, eating or drinking, smoking, or not wearing his or her seat belt.
Still, commercial driving is complex, perilous, and mentally taxing. People who drive as part of their profession are constantly having to juggle varying road conditions, traffic, cyclists, distracted pedestrians, other drivers, wandering animals, or sudden weather shifts. And they do so for hours at a time. Having MV+AI along for the ride promises to help drivers better navigate those complexities.
In the future, MV+AI technologies can also be brought to bear on workplace accidents, improving customer service, and smarter deployment of resources. For instance, you could gauge bus occupancy levels with machine vision, and use AI to dynamically calibrate levels of service according to shifts in demand. MV+AI can also be used to sense the load of a truck and adjust the engine torque, speed, and brake settings appropriately.
Getting there requires a tremendous amount of high quality information about the real world in order to train the algorithms needed to perform these advanced tasks. Lytx, for example, collects 50 billion pieces of data each day — from vehicle location and speed, to pedestrians and weather conditions. While much of the data are collected automatically via sensors, Lytx also deploys a team of professional reviewers who are trained to spot dozens of significant factors and conditions, adding a level of depth and quality to its data that’s invaluable from a research standpoint.
The ultimate goals of these endeavors are two-fold: to help fleets improve worker safety, and to advance operational efficiencies so they can thrive in today’s ultra-competitive environment. It’s a bold gambit, but one that could transform the entire industry.